Interpreting the links: causality and correlation to guide business
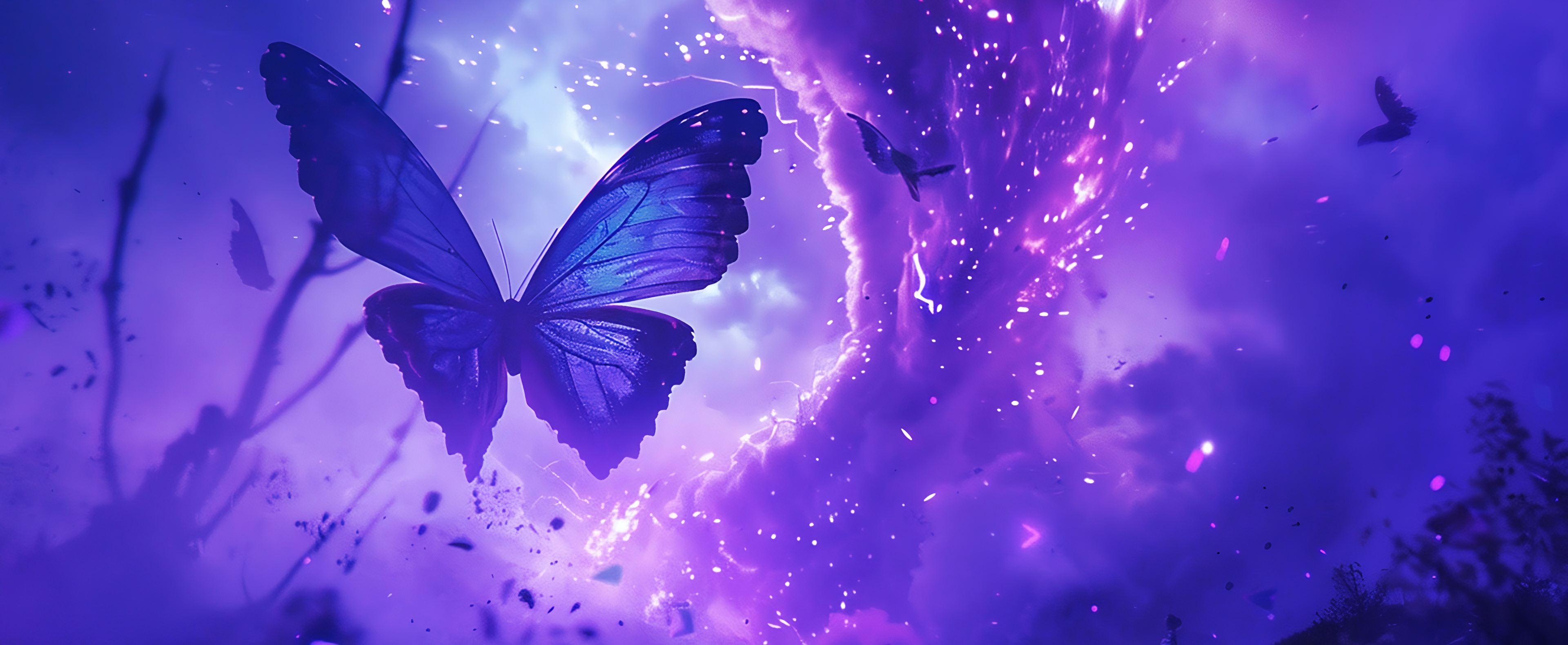
In 2012, the online advertising industry was worth $36 thousand million in the US alone, with the largest portion of revenue (46%) attributable to so-called 'search engine marketing' (SEM). Today the projections of global spending on advertising on digital channels arrive at more than 680 thousand million dollars (https://www.statista.com/outlook/dmo/digital-advertising/worldwide), so the data of 2012 seem negligible, but already at that time they were enough to push a team of eBay researchers to ask themselves if it were in some way possible to quantify the effective impact of these activities on the economic performance of the company.
Spoiler: yes. And Google didn't like it.
Analysing the data from a simple correlation between expenditure on SEM-type advertising and sales, the researchers first noticed a positive relationship: for every 10 per cent increase in advertising expenditure, there seemed to be a 9 per cent increase in sales: a result beyond all expectations and almost too good to be true. In fact, re-running the analysis taking into account control elements for geographical and temporal variability, the effect dropped to 1.3%. A greatly scaled-down result, but still positive. However, even this estimate did not take into account the major problem with an analysis of this type: namely that for advertising expenditure, in the specific context of SEM activities, the amount spent depends on the behaviour of the users doing a given browser search.
Economists and statisticians have a nice name for this problem, which represents one of the main challenges to the causal interpretation of a phenomenon: endogeneity. Simplifying greatly, endogeneity in an analytical problem occurs when one of the variables whose effect we want to measure ($ spent on advertising) on the target variable (sales) is correlated with something we do not see or know about, and which is also correlated with our target variable. In this applied case, we are talking about the user's intention or inclination to make a purchase on the eBay site at the time he or she does a browser search.
Spoiler No. 2: Keeping such a problem under control is possible, and we will shortly see how. In this specific case, the generalisable conclusion of the eBay researchers was that SEM activities have little or no impact, at least for recognised brands such as eBay, and that the correlation between clicks and purchases, identified as a measure of effectiveness by many advertising platforms, is not a reliable KPI for this type of activity. Excuse me? Wait, wait, I can already hear you shouting "Artificial Intelligence, Generative AI, LLM!".
Plot twist: at least for the moment, let's not talk about it.
In fact, now that the world has realised that 'Artificial Intelligence' is no longer just an expression from 80s sci-fi movies I would like to try to go further, by talking about something other than the umpteenth review of apocalyptic scenarios in which LLM or Computer Vision models threaten to substantially reduce the usefulness of certain occupations, if not render them totally superfluous.
To identify the correct causal links from the data so as to make the right decisions, AI is not the solution, but rather a tool that can be useful, but not decisive in itself.
To date, Artificial Intelligence (in which I also include the so-called traditional AI made up of the machine learning that has been used extensively by companies for a few decades now) has enabled companies to increase the efficiency and effectiveness of a number of activities. By this I certainly do not wish to diminish its importance and role, quite the contrary: just think of key processes for certain sectors such as insurance, where the combination of many small operational decisions (e.g. identifying which claims to watch out for in order to prevent fraud) is a central business advantage. Or, being able to identify which customers to contact for the success of a given sales campaign is vital for a retailer.
I would like to say, however, that while companies (or some of them) have so far been able to leverage effectively information assets to automate, speed up and improve the outcomes of a number of operational decisions, little has yet been achieved in the area of strategic decisions, for which, while there is no total rejection of reference to data, there is still a key to understanding missing: causality.
If in the training phase of a predictive model aimed at supporting operational decisions we can 'make do' (at least initially, gross of any fairness implications) with leveraging observed correlations, which may in some cases be spurious ("falsified"), in the case of models used to support strategic decisions, this is clearly not possible (or, if anything, strongly discouraged). Imagine deciding to invest millions on the basis of a correlation that later turns out to be spurious or, even worse, the result of reverse causation.
But let's go in order: what do we mean by causal interference or identification of causal relationship? For different degrees of depth:
- Testing whether or not a causal link exists in the process described by the data.
- Quantifying the causal effect.
- Understanding its actionability, i.e. how to act on this information.
Why is understanding the concept of causality so important for business? Applying in a hypothetical business context the three degrees above we could identify some questions:
"Did the sales campaign have an effect in terms of revenues?"; "What increase in redemption did the prioritisation of contacts bring about?"; "How much do I have to invest in activity X in order to get a certain return?"
.These are all seemingly simple questions, but more often than not, by answering them without paying attention to causal implications, the risk is to arrive at distorted answers, incorporating endogenous elements that bear no relation to the object of our question.
We need to start from a different assumption, another way of looking at the problem. We are helped by the fundamental concept of "counterfactual".
In order to correctly answer the question "Did the sales campaign have an effect in terms of revenue?" I should first be able to answer the question "What revenue would I have obtained if I had not done my sales campaign?"
.We need to compare revenues in the presence and absence of the commercial campaign (which, abstracting, we could call "treatment" or "intervention"). The point lies in the fact that, as the no-treatment state is typically unverified, precisely counterfactual, it is not immediate to reconstruct this estimate from the data.
There are two possibilities for obtaining this information. The first is to set up a real experiment. Still borrowing from a possible CRM setting related to a commercial campaign, this means allocating part of the population one would like to treat (the contact) to a control set. In this way, it will be possible to use the experience of this set a posteriori to construct a counterfactual estimate of what we want to verify and measure.
But if it's so easy, why isn't it always done?
Because it always involves a cost, and even when one is willing to bear it, it can be very complex to define the design of the experiment.
In the case of the sales campaign, the identification of a control sample implies ex-ante avoidance of potential sales that might result from the contact. In other cases, however, the 'treatment' whose impact is to be assessed may actually be the result of a combination of several factors, and defining an experiment to quantify the effects of each driver on the process may require a true design of experiments (DOE). In this case, think of a production process embodied in a recipe in which several levers of action are combined.
You simply cannot have discretion in the administration of upstream processing of a data collection, and must instead start with what you have.
And here is the second possibility. In these cases, there is usually no cost involved in identifying a control set, but, some extra energy will have to be expended in the analysis phase. Then one can try to use some identification strategies or modelling techniques to trace the available data back to a "quasi-experimental" context. Doing so means studying the processes underlying the data, their collection and the way in which the treatment(s) were administered in order to understand whether and how it is possible to trace them back to a context that allows us to estimate a counterfactual. This type of analysis is made possible by a series of tools and methodologies developed since the 1980s in areas such as econometrics and medical statistics. Methodologies such as Propensity Score Matching, Difference-in-Difference, and Regression Discontinuity Design, which have gradually incorporated some machine learning-derived techniques (especially to address dimensionality reduction problems).
Why aren't these approaches used and referred to more often? Because it is complex and expensive, and most often done for a "one-off" estimate. Or sadly, because reality may not necessarily be sweet. On the other hand, this one-off estimation would allow choices to be made in a robust manner and without fear of incorporating confounding factors.
In addition, at regular intervals we read articles and speeches explaining how progress is being made in considering causal logic in the world of Artificial Intelligence. Unfortunately, in the writer's experience, most of the time it is little more than the inclusion of some machine learning logic or modelling techniques within well-established causal inference frameworks. In other words, we could say that it is much more significant how much the academic world (traditionally attentive to causal inference issues) has managed to integrate from machine learning with respect to how much Artificial Intelligence has managed to integrate causal inference logic into itself.
I then call upon the AI Practitioners to call for a step change on these issues, so as to structurally integrate reasoning, techniques and mechanisms pertaining to the causal point of view into their models, since, for any business, the value of a correct and unbiased interpretation of its data and numbers is undeniable. And by this I also mean that we need to be ambassadors to our customers of such sensitivity and vision to accompany them in understanding approaches that can revolutionise their results.
Secondly, I urge companies not to be in a hurry to interpret the numbers, but rather to always ask themselves whether, in order to follow some trivial heuristic, they are not making overly strong assumptions that leave out latent distorting elements.
Slowing down, reflecting and looking at the same data with a critical eye and from a different point of view can save a lot of money in the long run, accurately quantifying the impact of a certain action so as to weigh up costs and benefits once and for all, or, again, planning specific interventions precisely tuned to the expected targets.
Author:
Giacomo Danda, Data Science Project Manager.